Streaming media is completely transforming the way we consume content. The growth in online streaming platforms is making it difficult for content providers to retain audiences. While on the user side, the problem of abundant choice is causing decision fatigue.
Enter: Streaming media recommendation engines. Serving content that resonates with user preferences - right from the start.
At its core, a media recommendation engine is an intelligent algorithm-driven system. It analyzes user data, establishes preferences, and studies behavior to curate personalized content recommendations.
But, the significance of streaming media recommendation engines extends beyond viewer satisfaction. For content providers, these engines can revolutionize the content strategy in unprecedented ways:
- They can better understand audience preferences and consumption patterns
- They can gather valuable insights into what works and what doesn't
- They can optimize content creation, acquisition, and distribution decisions
The end-goal? Enhanced success and profitability of platforms.
In this guide, we will explore the transformative potential of streaming media recommendation engines for your content strategy. Let's jump right in.
Maximizing User Engagement & Retention
Streaming media recommendation engines are sophisticated algorithms that analyze user data and behavior. These engines may use a mix of content-based filtering, collaborative filtering, and hybrid recommendations to deliver personalized recommendations.
By understanding individual preferences, these engines significantly enhance user engagement through:
Curated Content: Recommendation engines utilize a wealth of user data to understand individual tastes, such as:
- Viewing history
- Ratings
- Genre preferences
- Social interactions
And more. This tailored approach helps:
- users find content that resonates with them in a matter of seconds
- users spend more time on the platform, watching and discovering more content
- improve the user experience and lead to higher engagement
An AI-powered recommendation engine can compute preferences more accurately and quickly than traditional methods. This leads to improved user retention and positions streaming platforms to convert more casual users into regular viewers.
Use-Case: How Netflix Gets streaming Media Recommendation Engines Right
Netflix has had the first-mover advantage when it comes to personalized content recommendations. Here's what the brand gets right:
It uses a combination of:
Collaborative filtering: is based on the idea that people who have similar viewing history and preferences to you will have similar tastes. Collaborative filtering algorithms analyze data such as:
- What you've watched before
- What you've rated
- What you've shared on social media
- Your viewing history
Content-based filtering: focuses on analyzing the attributes and characteristics of the content itself, such as genre, actors, directors, and keywords, to understand the content's essence. By matching these attributes with user preferences, the engines can suggest similar content that aligns with users' interests. An excellent example of this is Netflix:
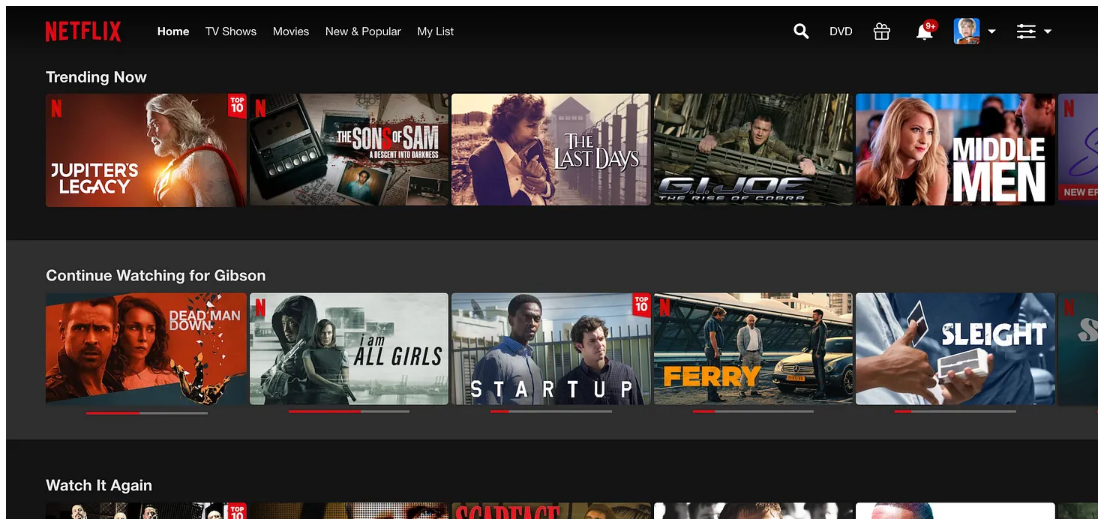
- Machine learning algorithms: take account of variables such as time of day, day of the week, and the user's location, to provide accurate and timely recommendations
- Netflix also uses A/B testing to analyze different algorithms and improve its recommendations continuously. For instance, here, Netflix tries to A/B test which of the "Stranger Things" artwork will attract the most number of plays by viewers:

Personalizing Content Discovery & Reshaping Content Strategies
New content discovery remains a stumbling block for viewers today. A majority still rely on commercials and word of mouth to inform the TV shows and movies they watch. Here lies the biggest opportunity for streaming giants to optimize their content strategy and boost content discovery for their subscribers with a recommendation system.
Considering that streaming media recommendation engines are powered by vast amounts of user data, content providers can easily help users find new content that is tailored to their unique interests.
Recommendation Engine-driven Content Discovery: What's In It for Content Providers?
Recommendation engines help:
Reinforce content discoverability: The vast libraries of streaming platforms can be overwhelming for users. Recommendation engines solve this problem by surfacing hidden gems and lesser-known titles that align with user interests. By expanding the content discovery process, these engines encourage users to explore and engage with a wider range of offerings.
Drive serendipitous discoveries: In addition to catering to user preferences, recommendation engines also introduce serendipitous discoveries. This includes:
- recommendations based on popular or trending content
- recommendations from influencers or experts
- curated playlists or collections
- customized genres or themes
Offering diverse and unexpected content suggestions keeps users engaged and excited about exploring new content.
3 Successful Content Discovery Strategies
1. Contextual Recommendations: use a variety of factors to suggest content that may be of interest to you based on your current context, such as the time of day, the device you're using, or your location.
For example, if you watch a lot of content on your phone in the evenings, the recommendation engine may suggest shorter, more digestible content that is suited to your viewing habits at that time of day.
2. Personalization: analyzes your viewing history and preferences and creates personalized recommendations that are tailored to you. The more data the recommendation engine has, the more accurate the recommendations will be.
3. The Long Tail: approach suggests recommendation engines should focus on recommending a wide range of niche content in addition to the most popular content. This approach recognizes that there is a large amount of content available and that individual viewers have widely varying tastes and preferences. By recommending a diverse range of content, recommendation engines can cater to all viewers and increase the likelihood that users will continue to use the platform into the future.
Improving Insights into User Behavior & Preferences
Let's now look at the data collection capabilities of streaming media recommendation engines that are fueled by large user-centric datasets such as:
- Viewing History to capture details about the content users have consumed, how long they watched it, and patterns in their viewing habits
- Ratings and Reviews to gather valuable feedback on content quality and preferences, understand user sentiment and incorporate it into the algorithms
- Interaction Data such as rewinding, fast-forwarding, pausing, or skipping content to access insights into user engagement levels and preferences within specific scenes or moments
- Demographic Information such as age, gender, location, and language preferences to understand the requirements of a diverse user base and tailor content accordingly
How Content Providers Can Use This Data to Improve Their Offerings
The data collected by streaming media recommendation engines holds immense potential for content providers to enhance their offerings. Here's how:
Identify popular content and improve content search: By analyzing viewing data and data from user search queries, providers can:
- Identify popular programs and genres that are in high demand and use this information to guide content acquisition and creation decisions
- Identify search terms that are commonly used and improve search functionality accordingly
This data can help identify gaps in the content library, single out emerging trends, and enable content providers to invest in content that resonates with their target audience.
Optimize content delivery: Providers can use data on how users interact with their content to identify areas where delivery can be optimized, such as by improving streaming quality or reducing buffering times. Plus, user data can reveal patterns in user engagement.
Use-Case: Spotify Uses Data to Improve Content Recommendation to Users
Spotify uses data on user listening habits, playlists, and social interactions to curate personalized music recommendations:
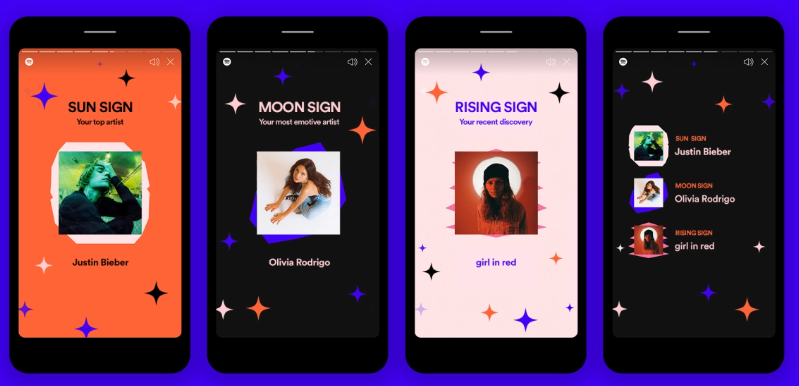
This data-driven approach has helped the platform enhance user satisfaction and keep users engaged with personalized music discovery.
Increased Monetization Opportunities
If implemented correctly, streaming media recommendation engines can offer multiple opportunities to drive conversions and increase revenue for content providers:
- Upselling and cross-selling by suggesting premium or add-on content options to users based on their preferences and viewing history
- Promoting exclusive content/limited-time offerings in partnerships with other brands to users who have demonstrated interest in related content
- Providing dynamic pricing and offers based on user preferences, historical purchase data, and market trends
- Offering personalized content and targeted advertising that viewers are most likely to enjoy and hence, boosts the time spent viewing content, which can lead to more chances for ad clicks and subscriptions or in-app purchases
4 Successful Monetization Strategies & Important Lessons
1. Amazon Prime Video leverages data on what users are watching and how they interact with content to inform their content creation and acquisition decisions.
For example, they have developed a number of original series, such as "The Marvelous Mrs. Maisel," that have been praised for their unique and engaging content. Plus, these shows have helped garner 5 million new members:

Amazon's "Recommended for You" feature also provides a list of TV shows and movies that users will likely enjoy based on their viewing history.
By understanding user preferences across its e-commerce and video streaming platforms, Amazon is able to deliver a seamless and personalized user experience.
2. Disney+: Disney+ utilizes a recommendation engine to offer personalized suggestions and leverage its extensive content library. By bundling its streaming service with other offerings like Hulu and ESPN+, Disney maximizes revenue through a multi-platform strategy and increases user engagement.
3. YouTube Premium: YouTube's recommendation engine also drives conversions by promoting YouTube Premium, a subscription service that provides ad-free viewing, exclusive content, and offline access:

YouTube effectively monetizes its platform by strategically showcasing the benefits of premium membership via personalized recommendations.
4. HBO Max: HBO Max leverages its recommendation engine to upsell users to premium tiers or promote content from partners and affiliates. By delivering targeted suggestions based on user preferences, HBO Max increases revenue by enticing users to explore and engage with premium offerings.
Success with streaming Media Recommendation Engines
Long story short, an effective content strategy must now include the use of recommendation engines to prioritize personalization, data-driven insights, and user-centric experiences.
Using this advanced functionality, content providers can:
- maximize user engagement and retention
- reduce decision fatigue and churn rate
- enhance the viewing experience
- foster long-term relevance
These benefits are crucial for content providers looking to stay competitive in an increasingly crowded market.
As technology continues to advance, these engines will become more sophisticated in their ability to personalize content recommendations. This means that content providers who adopt streaming media recommendation engines early on will have a significant advantage over their competitors.
By doing so, they will not only provide a more tailored viewing experience for their audience but also unlock the full potential of content offerings while keeping viewers coming back for more.
How does the Argoid streaming Recommendation Engine Work?
The Argoid streaming Recommendation Engine is an AI-driven personalization engine that offers hyper-personalized recommendations to every user across touchpoints and in real-time.
The AI engine uses various types of data, such as user profile, behavior, preferences, intent, context, and content metadata, to match the user with the most relevant and accurate content.
The engine also adapts to the user’s changing interests and feedback, predicting what the user may want right at the moment they want it.
The Argoid streaming Recommendation Engine uses the strength of AI and data to help streaming and streaming platforms optimize their content strategy, as well as improve business outcomes.