Recommendation engines are all around us.
You have Amazon suggesting products that you can buy based on your browsing or purchase history. Similarly, Netflix tells you which movies or shows to watch depending on the kind of content that you consume. Facebook recommends friends, while YouTube offers you videos based on your interests, and Tinder shares potential dates depending on your profile.
These use cases are prime examples of how recommendation engines can boost a business’s bottom line. Studies have consistently demonstrated how recommendation engines are doing a stellar job of enhancing customer experience, boosting conversion rates (by 288%), increasing the average order value (by 369%), preventing cart abandonment (by 4.35%), and driving engagement.
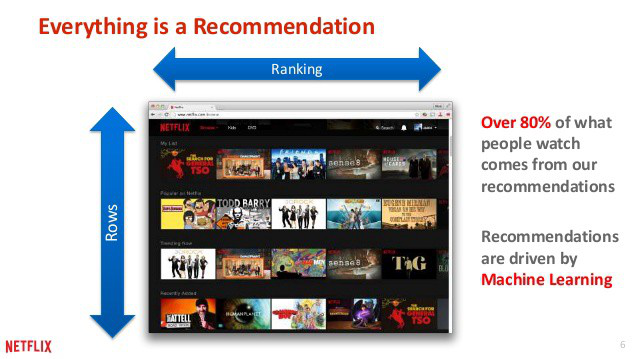
However, like every piece of digital technology, not all recommendation engines were created alike. Here, we will be reviewing the different types of recommendation engines and find out which one is the best.
What are the Different Types of Recommendation Engines?
The different types of recommendation engines can be broadly categorized on the basis of the technique or the technology behind them. We have elaborated on these in the following sections.
Types of Recommendation Engines Based on Technique
Recommendation engine can be divided on the basis of the logic that governs the flow and outcomes. Accordingly, here are some common types of recommendation engines based on technique:
1. Rule-Based Recommendations
Rule-based recommendations are the most simplistic and basic forms of recommendation engines. You need to define the rules, and as the name indicates, the recommender system will make recommendations when the rules are satisfied.
Say you establish a rule stating if a person is interested in remotes, they would be interested in batteries too. Accordingly, anybody who shows an interest in remotes will be recommended batteries.
2. Segmentation-Based Recommendations
To perform segmentation-based recommendations, you first need to understand the concept of customer segmentation. Customer segmentation is typically a marketing process that divides the customer base into subgroups depending on factors such as age, gender, location, and other demographics.
Since this bunching is performed on the basis of a few commonalities, it lays the foundation for the intuitive assumption that if someone from group A is interested in item X, then maybe another individual from group A could be interested in X. For instance, a user from Mumbai looks up an umbrella in June. Your recommendation engine can learn from this and suggest umbrellas to other shoppers from Mumbai when they visit your store in June.
3. Configuration-Based Recommendations
Configuration-based recommendations are based on multi-attribute value theory. Here, the priori value functions and attributes (called criteria) are assigned weights, and products are recommended in the order of decreasing weights. In some instances, these products may be tabulated and compared to highlight variations.
Suppose someone wants to buy a smartphone. For them, the RAM, internal storage, and brand are the three criteria that matter. Suppose they input brand these details as 4GB, 64GB, and Apple, then the recommender may suggest iPhones 12, 11, SE, that fit the configuration.
Types of Recommendation Engines Based on Technology
The advancements in artificial intelligence (AI) and machine learning (ML) are making recommendation engines smarter. Based on such technologies, these recommendation engines can be classified as:
1. Collaborative Filtering
Collaborative filtering (a.k.a., Social Recommender systems) is one of the most common engines that power various websites. Such recommendation engines analyze user behavior, preferences, activities, etc. to predict their likes or dislikes, depending on the insights drawn from similar users, so as to make relevant suggestions.
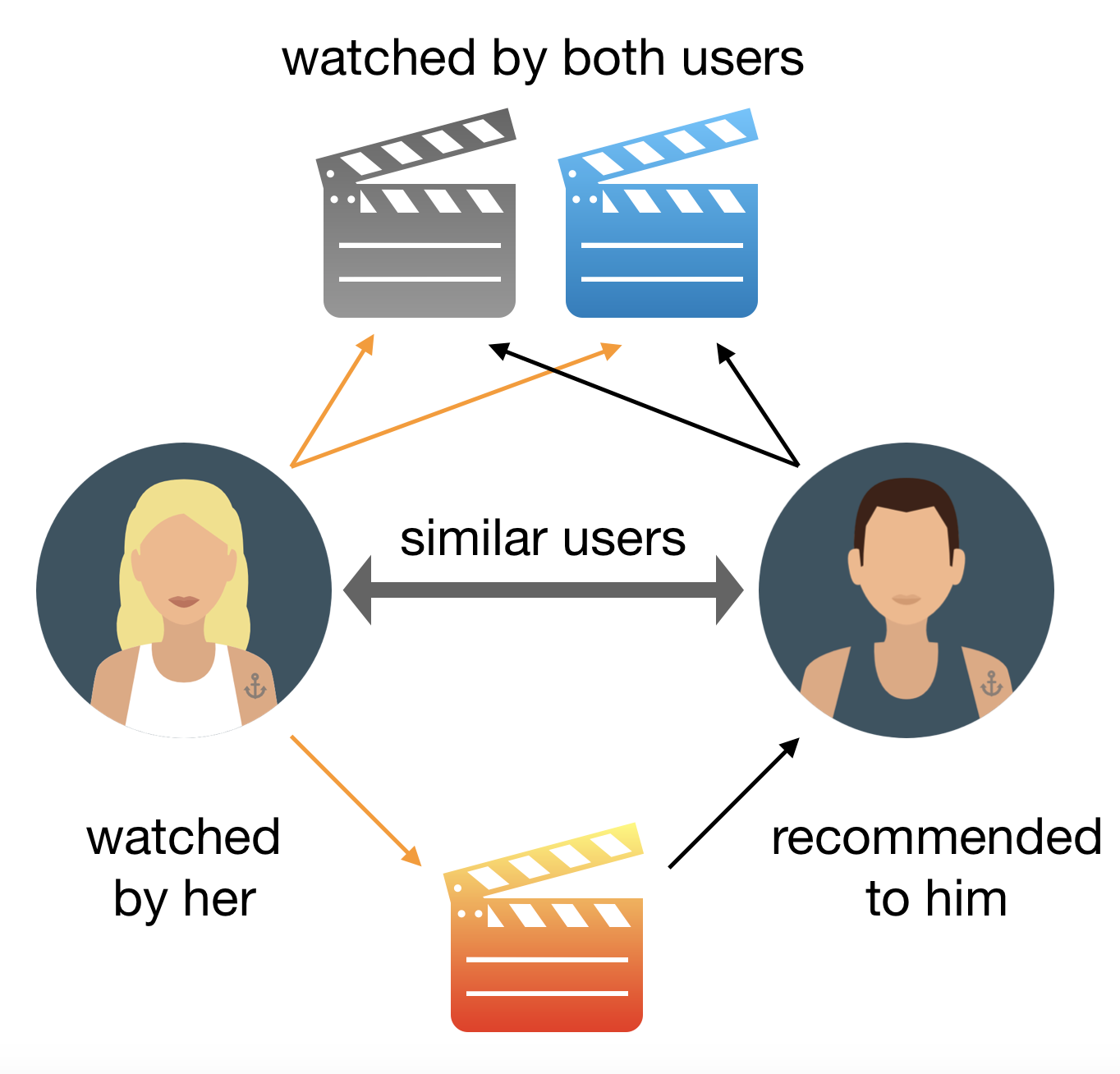
Collaborative filtering can be user-to-user recommendations (a.k.a., user-based collaborative filtering) or item-to-item recommendations (item-based collaborative filtering). In both cases, the content is beyond the purview of the machine analyzable content. Hence, the recommendation engine may not even be cognizable of what it recommends!
2. Content-Based Filtering

As the name indicates, content-based filtering depends on the description of the product or the user. Here, in the content-based filtering generates a profile for the user and the product, which serve as the attributes for comparison. Then, depending on the keywords, the recommendation engine correlates the product and the profile through a similarity score, which assigns a priority to the product. Finally, the product is displayed to the user based on the weighted score and priority.
3. Hybrid Recommendation Systems
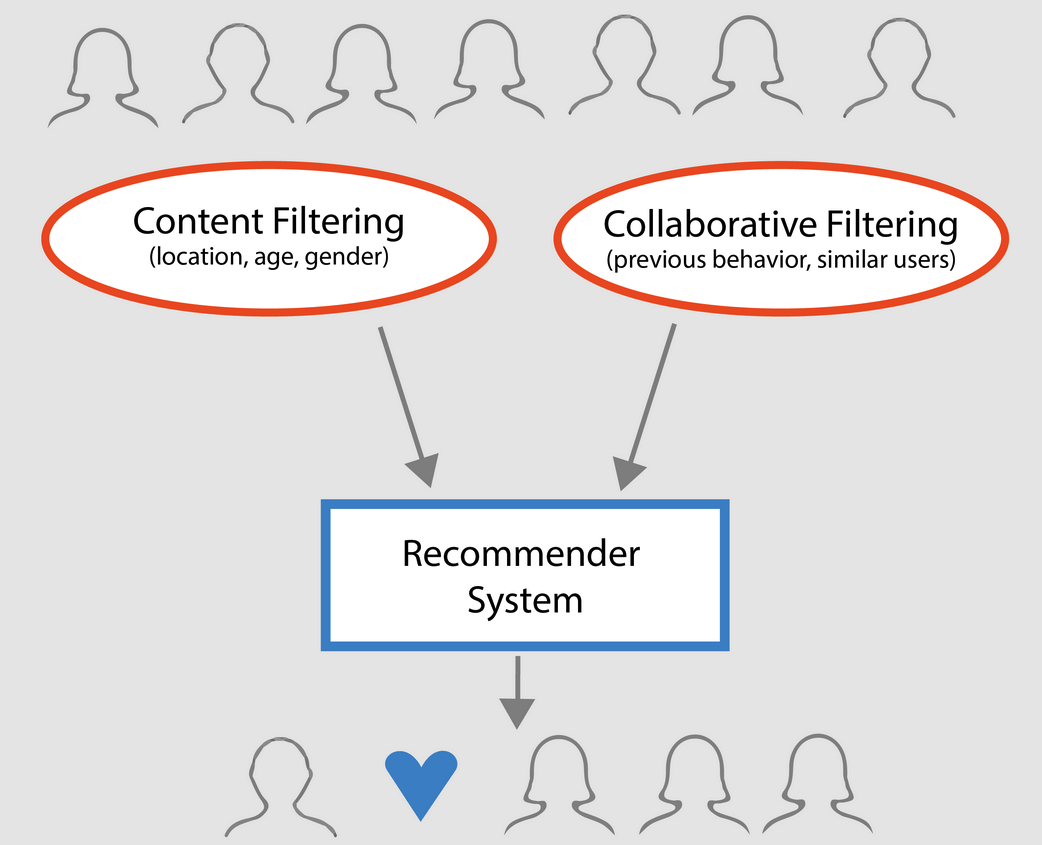
Hybrid recommendation systems combine collaborative (transactional) and content-based (meta) filtering to make the recommendation engine more effective. The combination of the two mitigates the drawbacks and amplifies the benefits of the pure approaches.
Hybrid recommendation systems combine collaborative (transactional) and content-based (meta) filtering to make the recommendation engine more effective. The combination of the two mitigates the drawbacks and amplifies the benefits of the pure approaches.
Netflix is a shining example of a hybrid recommender system that uses collaborative filtering (by processing the searching or viewing habits of the user) and content-based filtering (by suggesting content that shares common characteristics with what they have recently watched or rated).
Comparison of Recommendation Engines That Use Different Technologies
As we are focusing on the latter type of recommendation engines, let us review these through a detailed comparison:
Which is the best?
Even though each type of recommendation engine has its fallacies just as it has its high points, no single solution can serve as a silver bullet to cover all scenarios.
In some instances, you might find rule-based recommender systems to be easier to implement, while content-based filtering would be better equipped at catering to customer requirements. The success of a hybrid recommendation system is a testament to this fact. However, even the hybrid recommendation engine is not an end-all, be-all solution.
Recommendations need to be a complementary effort. As a result, rather than focusing on a single procedure, businesses can maximize the effectiveness through a dynamic algorithm that hand-picks the recommendation engine framework depending on the situation.
Fortunately for you, Argoid is such a magic pill that can scale according to these changing conditions. How do we do it? And how can it help you? Contact us to find out