On-demand streaming services offer us almost unlimited content for an affordable monthly subscription. Streaming services have grown to become a big part of our social life. These services are a catalog of relatable content and endless topics of debate. It has now created a pivotal impact on our lifestyle.
The influence that these services have in our lives is imminent from tiny nuances and cultural references and the cult following that TV shows and movies gather. OTT Streaming services have evolved mainstream cinema and television, bringing in an ever-increasing user base into its garb.
The growth of subscription-based on-demand streaming services is imminent from data published by them, with Netflix adding as many subscribers in a quarter as they did in the year 2019. With work from home cutting down on work commute times, there is now a lot more time that can be spent in leisure than ever, not to mention that such a paradigm shift has allowed for more family time to bring them closer. Many streaming services, especially Netflix top-notch video personalization platform has added as many as 50–60 new movies a month and are increasingly making family-oriented content.
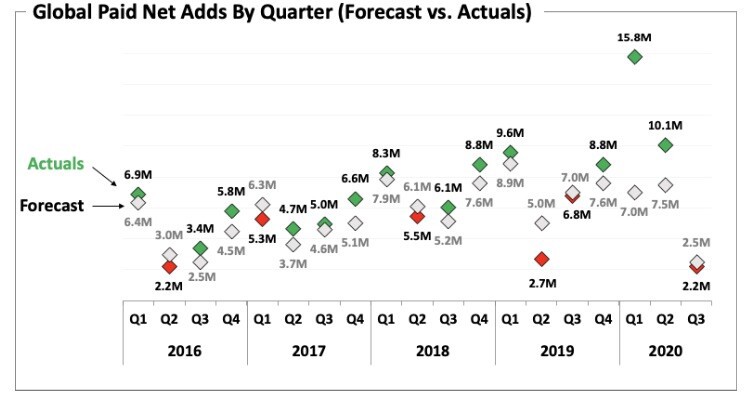

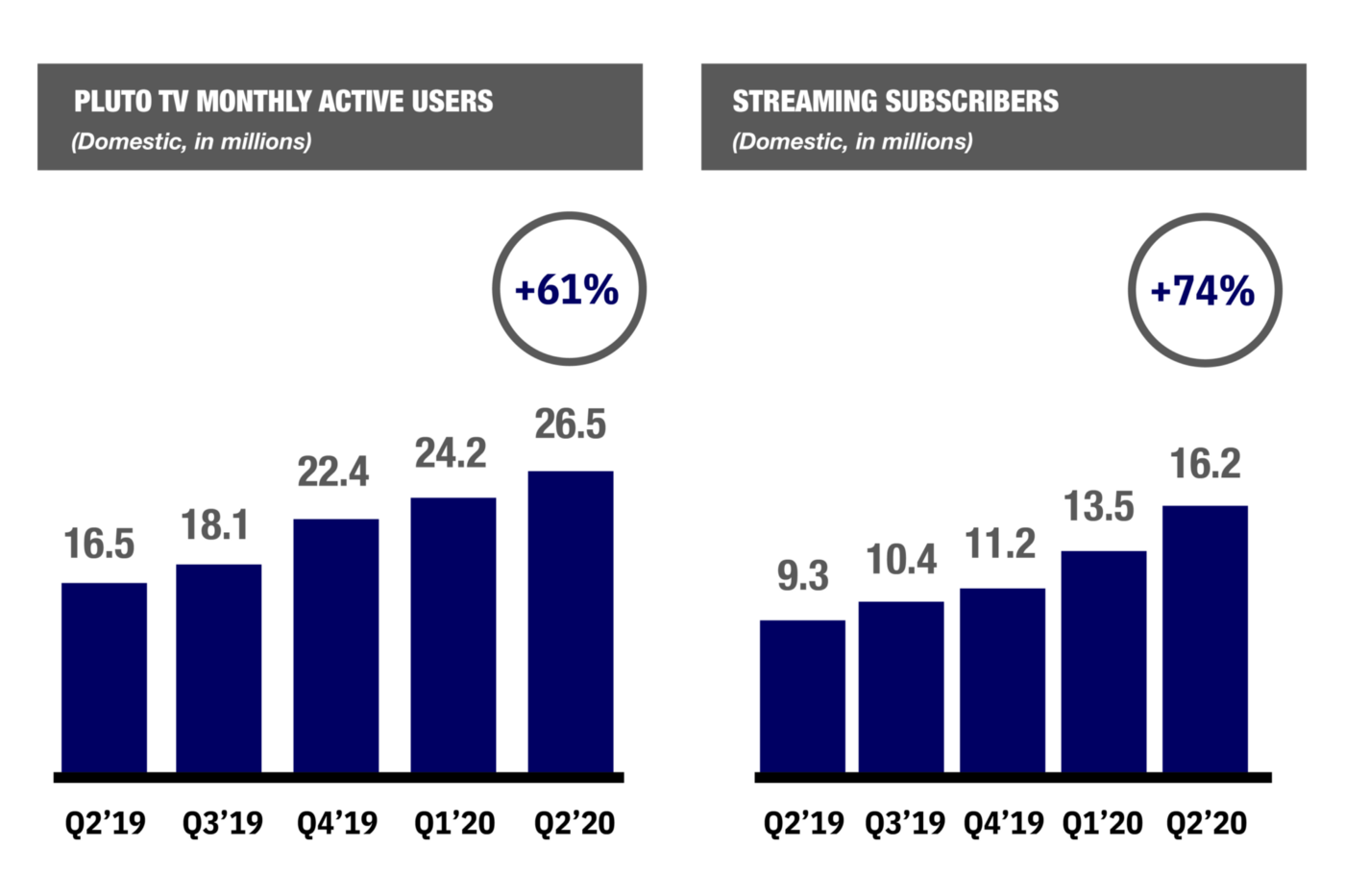
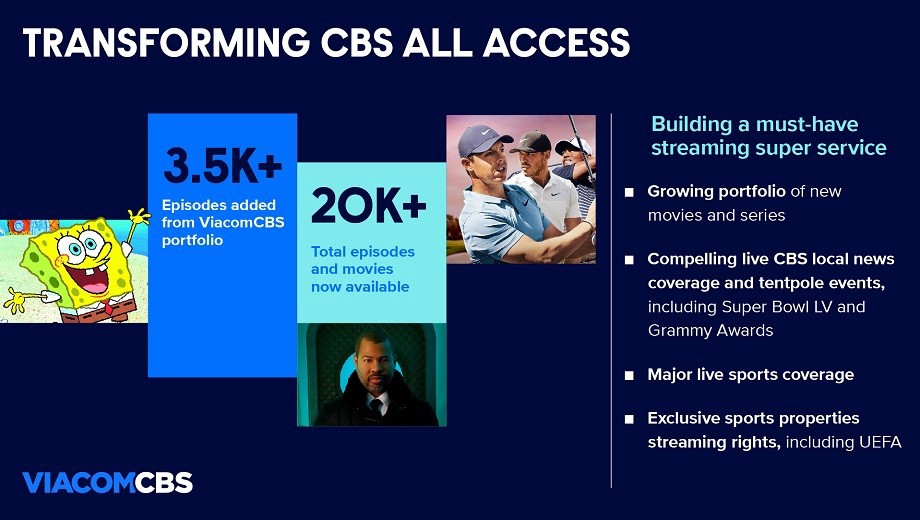
This influx of content and subscribers means that there is now more content available to an average subscriber than they can watch in several lifetimes. In an age where digital media is constantly bombarding our devices, how do we find that one telecast that we shall remember as a mind-numbing transcendental shift in our perspective? Or, how shall content creators tailor such experiences, in an era of ratings and its stronghold on loyalty and disdain?
Humans can process only a few pieces of information at a time. However, streaming services typically have thousands of shows to watch from and at least 20–30 shows per page on their site. So, even if a user gives 15 minutes of her time looking for content, she can only spend 30–45 seconds evaluating a telecast before deciding on what she would want to watch. The front page of a platform is a marketing page, which sets a precedent for what essentially is a gamble for watch-time. It could end up with a user spending their weekend breaks on the platform or them moving on to another.
Providing Recommendations to the User
A platform needs to provide content that aligns with the preferences and interests of users. Often, a simple questionnaire or profile page can get the preferred genre, cast, or language. But more often than not, users show seemingly unpredictable streaming patterns, which a survey may not capture. As the tastes of users differ from one another, across different demographics and personalities, compounded by cultural differences, recommendations can quickly become a complex problem encompassing many parameters.
A Recommendation Engine would, in short, require a system that interfaces with existing content, which in the case of a streaming service, are the streaming titles, or algorithms that prioritize content based on parameters.
Data Acquisition for Recommendation Systems
1. User Profile Data
Video-on-demand services can use profile data and session files to allow users a wide array of features, such as web-caching, smart suggestions, and offline playback. Systems use cookies, storage APIs, and device identification methods to analyze user preferences and provide them with device and region-specific content.
User profile data would, in general, include details such as user preferences, region, the device used, and purchase history.
2. User Interaction Data
User Interaction Data is composed of user watch history, search terms, and can include comments or ratings the user has given certain content.
3. Video/Streaming Content
Video/Streaming Content includes video metadata, codecs used, and video files. These are usually stored in databases and may include reference links to external databases.
Data Preprocessing
User tracking data must first be organized and cleaned of any noise (or any external inconsistencies). Cleaning involves removing redundancies in data, checking for the structural integrity of the data, such as by correcting naming conventions, and finally going in for data verification with known parameters to ensure proper collection of data.
Data Cleansing
User data may contain inaccuracies due to formatting errors or missing values. Other strategies are scaling and normalization of data, appending character encodings, and preliminary parsing of data such as dates, strings, etc. [4]
Data Enrichment
Data that is present in a system may need augmentation from multiple reference sources. A good example is a movie catalog on a website, which may require additional information such as cast, rating, or release date from public databases or review sites. Further, databases may need to update data regarding ratings, accolades, etc. Data Enrichment deals with systems that consolidate data from different databases to complete missing or incomplete data or further enhance data for its usability and processing. [5]
Recommendation Engines for Smart Recommendations
Candidate Generation
Here, the engine searches for content that is relevant to the query. An example of this is if a user watches two videos on a particular topic, such as houses, the engine searches for videos on architecture or housing. Another criterion could be using a collaborative recommendation engine. Here, relevant content is suggested based on the browsing habits of constituent users in a user-group, made based on user profile and pattern recognition. So, if a user in a certain user-group watches a video, the same video is suggested to users who are in the same user group.
Recommendation systems provide candidates based on a query. A query can either be user preferences, similar content, or a host of other factors. Recommendation systems are divided based on the type of algorithm for candidate generation.
Demographic Based Recommender System
A quick solution could be to isolate the demographic of the user base and suggest to them content that is popular in the region. But this could be inaccurate when considering that most locations provide habitation for a cosmopolitan and cultural divide and that not everyone may have the same personality. Such a cultural distinction is prevalent in major countries like India, the USA, and Japan, where there are many different cultures of people residing there.
Content that is popular, or watched by many, may not serve as indicators of relevance. since a TV premiere or movie that is trending on streaming platforms may not appeal to the user. After all, we can all agree that we often look past the popular or trending in your region pages on YouTube. Such recommendation engines prevent new content from breaking in and enjoying a following since no user may have been exposed to that content yet.
Knowledge-based Recommendation System
Another approach could be to suggest content based on certain characteristic features, such as ratings, awards, or the cast in general. Most streaming services actively promote such content to the user in the form of exclusives or premieres. However, not every user shares the same interests as the average user and excludes the influence of outliers. IMDb listings are a great example, where there is no strong correlation between the popularity of a series and its rating.
Content-Based Recommendation System
This type of content-based recommendation is quite popular and has its roots in social media, where users would fill out their interests, favorite events, music, etc. The platform would then use this to recommend acquaintances, events, and show advertisements. These types of recommendation systems use contextual information from the user to target them with content based on ranking and scoring algorithms.
Hybrid Recommendations
Frequently, these recommendation systems can work in combination with each other. Most streaming platforms provide popular trending pages, along with genre-based recommendations, as well as a hybrid between ‘popular’ and ‘based on what you like’ content.
Ranking
The recommendation system ranks the chosen content based on their order of similarity and relevance. The system ranks the candidates based on characteristic features. The system then draws a mathematical analogy between these features based on the similarity of one candidate to another and calculates an analogical distance between them. So, consecutive cat videos would rank nearer and hence, be more identical than a cat and a dog video.
Scoring
The system uses a set of objectives to score the content. These objectives could be either to maximize the click-through rate, maximize watch-time, or in some cases, even to maximize ratings for a piece of content. Here, many factors are taken into consideration when scoring. For example, a recommendation system places the objects on the screen only after the ranking and scoring process is over. However, the position of objects on the screen may introduce a bias into the system since users are more prone to click items on the top of the screen than the bottom. Hence, recommendation systems have to factor the above in the scoring process itself.
Re-Ranking
Here, the algorithm ranks content after the scoring process again based on criteria, which may change the type of candidates generated. For example, a query for a mezzanine cat would eliminate candidates for the search query cat because of the filter mezzanine. The recommendation system then narrows the pool of candidates and subsequently affects the scoring and ranking process.
Recommending content based on the system of modeling for watch time has helped video streaming services like YouTube improve their prediction on user analytics better than traditional methods for fitting data. Including algorithms that are more inclusive of features, such as the age of the content, allows for a better ranking of popular videos. These recommendation engines also power Google Analytics and Ad words, which have proven to give valuable insights on how content creators may make series or movies that would better suit their target audience. [6]
Using AI/ML for Better Recommendations and Comprehensive Video Analytics
Recommendation systems provide users with content that aligns best with their interests. These systems are pivotal to the success of platforms such as video-sharing platforms like TikTok, Youtube, social media sites like Instagram and Twitter, and streaming services like Netflix, Hulu, and Prime Video.
Such systems have shown moderate success in suggesting content based on matching search queries, keywords and ratings. However, telecasts are more than just ratings and comments on a dashboard. Movies or TV series have a premise, plot, and context. Simple recommendation engines only provide keyword-based matching, which leaves many distinctive features out of the dataset.
Modern recommendation systems, hence, analyze catalog by its thumbnail or artwork, closed captions, and even movie trailers. Machine learning techniques such as natural language processing provide tools for comprehensive text analysis and context interpretation that easily integrate into conventional machine learning paradigms. [7]
Techniques such as Deep Learning and Neural Networks provide tools to further analyze data points and use probability and statistics for better scoring and candidate generation. Reinforcement Learning is another method of machine learning that uses penalties and rewards for better scoring and candidate generation.
Recommendation systems that use these techniques are available through APIs that provide users with easy integration of these techniques without having to worry about the inner workings of these systems.
How Argoid Can Leverage Trends in AI To Provide Personalized Recommendations
Argoid provides customers with API driven analytical tools for AI-based Personalization at every touchpoint. It leverages leading trends in machine learning to offer businesses insights into consumer analytics and provides seamless integration into existing platforms.
Argoid provides real-time personalization (Real-time Relevance) through its differentiated technology:
- Automated Data Curation — Argoid provides solutions for real-time and batch processing of data aggregated from different user events and make it available for the recommendation engine.
- Data Optimization — Argoid provides autonomous and scalable data processing systems for machine learning models. It does this by downsampling data without loss of information.
- NLP/Text Processing — Argoid provides text processing and extraction pipelines, using natural language processing for better predictive analytics.
- Auto ML — Argoid evaluates data against multiple machine learning models in real-time and obtains the best fit for a particular use case, all without requiring a dedicated team of data scientists.
- Machine Learning Model Catalog — Argoid provides a vast collection of machine learning models for a wide variety of use cases, to ensure the use of an appropriate model for a given application. Argoid’s machine learning models make the best use of available resources and multiple pipelines to provide a scalable infrastructure for machine learning and analytics.
- Cutting-Edge Graph Engine — Argoid has built a a graph engine that supports a whole variety of use cases, including data enrichment, autonomous clustering, and cluster labeling. Argoid’s solutions provide built-in support and seamless integration with its graph engine.
- Big Data Stack — Argoid provides a reliable, scalable, and fault-tolerant tech stack that can be run on-demand.
- Configurable Engine — Argoid provides a configurable engine for extensive customization of use cases to suit the needs of every service or platform.
Using Argoid to Power Cloud-Based Recommendation Engines
Argoid provides end-to-end solutions for businesses to deploy infrastructure for smart search, recommendations and customer analytics in the cloud.
Integration with Cloud Computing Platforms
Services can integrate Argoid’s recommendation engines into existing infrastructure such as Google Cloud Platform, Amazon Web Services and Azure. Argoid’s recommendation engines are cloud-agnostic and can be deployed on-site.
Intelligent Recommendation Models
Web services may choose from a wide array of recommendation systems and use customized machine learning models to provide users with relevant content.
Data Analytics and Feedback
Make use of big data analytics to provide users with comprehensive reports and valuable feedback.
FAQ's on personalization in OTT space
How can AI help for better product recommendations?
An artificial intelligence recommendation system is a class of algorithms that offer relevant recommendations to customers. AI helps ensure that products are suggested to the customers according to their intent. Artificial Intelligence allows brands to highlight the products other customers liked in front of new customers.
What are cloud-based recommendation systems?
When a cloud user requires suggestions for cloud service, a cloud based recommendation system determines an automatic service which is based on the previous interactions by the user.
What kind of customer data is required for leveraging personalized recommendations?
Customer data to analyze user preferences and leverage recommendations are region, the device used, purchase history and so on. User Interaction Data is also required composed of user watch history, search terms, ratings the user has given certain content. Video/Streaming Content used are video metadata, codecs used, and video files. User data may definitely contain inaccuracies due to formatting errors etc. There are strategies like scaling and normalization of data, appending character encodings, etc.